What is a Meta Analysis in Research with examples
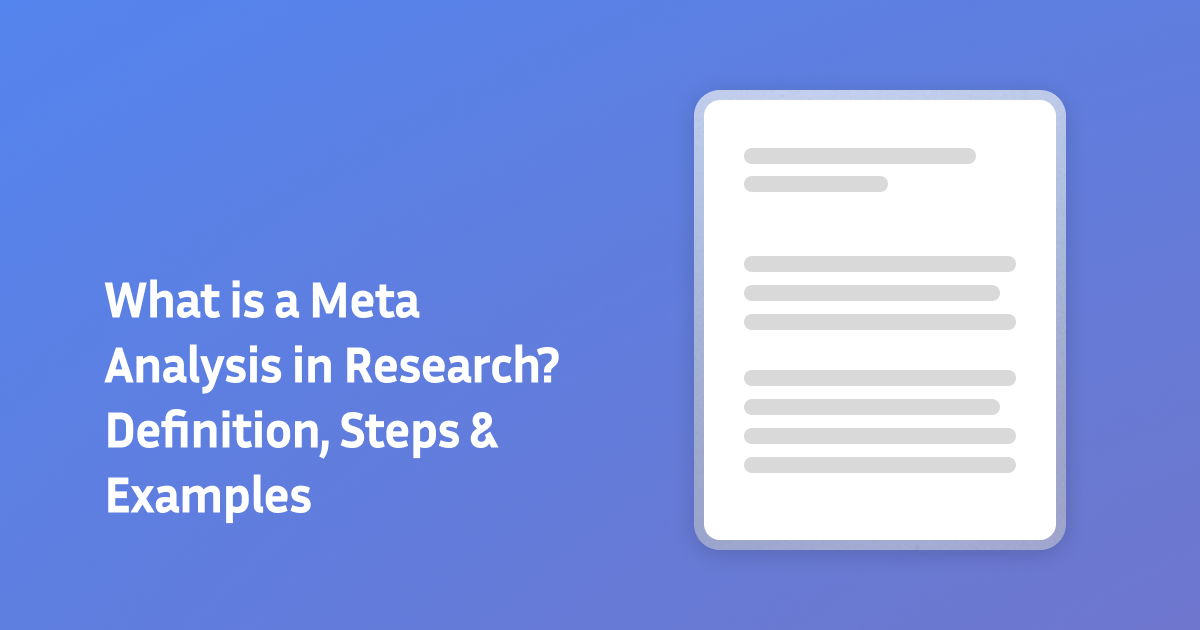
When researchers want to go beyond simply collecting studies and actually quantify the collective findings, they turn to meta-analysis. Think of it as a statistical microscope that can zoom in on research results, transforming individual study outcomes into a powerful, numerical understanding of scientific evidence. Meta-analysis doesn't just summarize research – it mathematically combines and analyzes results to reveal deeper, more precise insights.
What Exactly is a Meta-Analysis?
A meta-analysis is like a detective's ultimate tool for understanding research. It's a sophisticated statistical method that combines and analyzes results from multiple scientific studies on the same topic.[1] Think of it as a research superhero that can:
- Synthesize findings from numerous individual studies
- Provide a more robust and comprehensive understanding
- Identify patterns that might be hidden in single research projects
- Increase the statistical power of research conclusions

Feeling overwhelmed by the complexities of meta-analysis? Try Paperguide, the all-in-one AI research assistant designed to transform your research process. Whether you're conducting a meta-analysis, analyzing papers, or synthesizing complex research, it streamlines every research step.
Why Meta-Analyses Matter
Let's break down why meta-analyses are so incredibly valuable:
1. Seeing the Bigger Picture
Imagine trying to understand a complex topic by reading just one book. Now imagine reading 50 books on the same subject. That's the power of a meta-analysis – it helps researchers see beyond the limitations of individual studies.[2]
2. Increasing Research Reliability
By combining multiple studies, meta-analyses can:
- Reduce the impact of random errors [3]
- Provide more statistically significant results
- Offer more reliable and generalized conclusions [3]
3. Revealing Hidden Insights
Sometimes, individual studies might seem contradictory. A meta-analysis can help:
- Identify consistent patterns
- Explain why different studies might have different results
- Uncover nuanced insights that single studies might miss
Types of Meta-Analysis
Quantitative Meta-Analysis
Statistically combines numerical data from multiple studies to estimate an overall effect size.
Qualitative Meta-Synthesis
Integrates themes and concepts across qualitative studies to generate new insights or theories.
Bayesian Meta-Analysis
Incorporates prior knowledge and uncertainty using Bayesian statistics to update probabilities based on new evidence.
How to Define a Research Question for Meta-Analysis
A strong research question is:
- Specific: Clearly defines the population, intervention, comparison, and outcome (PICO)
- Focused: Avoids being overly broad or vague
- Feasible: Relies on accessible, quality data
- Meaningful: Addresses a real gap in the literature
Example: What is the effect of cognitive-behavioral therapy (CBT) on reducing social anxiety in adolescents?
How AI Tools Can Simplify Meta-Analysis
AI tools like Paperguide streamline the process by:
- Automating literature searches across multiple databases
- Screening studies using machine learning
- Extracting data from PDFs and structuring it into tables
- Performing statistical analyses and generating forest plots
- Producing PRISMA-aligned reports
Paperguide Insight: AI helps reduce workload by up to 70%, allowing researchers to focus on interpretation and strategy
How a Meta-Analysis Works: A Step-by-Step Journey
Step 1: Defining the Research Question
Researchers start by clearly defining what they want to investigate. This is like setting a GPS coordinates for their research journey.
Step 2: Systematic Literature Search
- Collect ALL relevant studies on the topic
- Use strict inclusion and exclusion criteria
- Ensure a comprehensive and unbiased search
Step 3: Evaluating Study Quality
Not all studies are created equal. Researchers carefully assess:
- Research methodology
- Sample size
- Potential biases
- Relevance to the research question
Step 4: Data Extraction and Statistical Analysis
- Extract key data from each study
- Use advanced statistical techniques to combine results [4]
- Calculate effect sizes and statistical significance [4]
Step 5: Interpretation and Reporting
- Synthesize findings
- Discuss implications
- Highlight limitations and potential future research directions

Real-World Examples to Bring Meta-Analysis to Life
Medical Research
- A meta-analysis might combine studies about a new medical treatment to determine its overall effectiveness across different patient groups.
- Investigating the cumulative effectiveness of various interventions for managing chronic pain.
- Examining the long-term impacts of specific vaccination strategies across multiple population groups.
Psychological Studies
- Researchers might use meta-analysis to understand the impact of a specific therapy approach by analyzing multiple clinical studies.
- Exploring the effectiveness of mindfulness interventions on reducing anxiety and depression.
- Analyzing the cognitive impacts of sleep deprivation across different age groups and research contexts.
Environmental Science
- A meta-analysis could examine climate change effects by synthesizing research from various geographical regions and research teams.
- Investigating the impact of renewable energy technologies on carbon emission reductions.
- Assessing the effectiveness of various conservation strategies on biodiversity preservation across different ecosystems.
Challenges and Limitations of Meta-Analysis
Meta-analyses, despite their power, face several significant challenges:
1. Quality of Included Studies
- Not all studies are created equal
- Varying methodological rigor can skew overall results
- Researchers must carefully evaluate and potentially exclude low-quality studies
2. Publication Bias
- Positive or significant results are more likely to be published
- Negative or inconclusive findings often remain unpublished
- This can lead to an overestimation of effect sizes or skewed conclusions [5]
3. Methodological Complexities
- Requires advanced statistical expertise
- Choosing appropriate statistical methods is crucial
- Complex decisions about how to combine studies can impact results
4. Heterogeneity Challenges
- Studies may differ in Population characteristics, Research methodologies, Measurement techniques, and Contextual factors. [6]
- These differences can make direct comparisons difficult
5. Potential for Oversimplification
- Risk of reducing complex findings to a single summary statistic
- May miss nuanced insights from individual studies
- Can potentially overlook important contextual variations
6. Data Limitations
- Dependent on available published research
- May be constrained by limited or incomplete data
- Quality of meta-analysis directly reflects available studies
When to Use a Meta-Analysis: A Comprehensive Guide
Ideal Scenarios for Meta-Analysis
- Multiple Studies Available
- At least 5-10 high-quality studies on the topic
- Studies show varying or inconsistent results
- Desire for a comprehensive understanding
- Research Synthesis Needs
- Seeking to establish a definitive conclusion
- Wanting to understand overall trends
- Need to resolve conflicting research findings
- Specific Research Contexts
- Medical and health research
- Psychological interventions
- Educational effectiveness studies
- Social science investigations
- Environmental and climate research
Red Flags: When to Avoid Meta-Analysis
- Too few studies available
- Extremely heterogeneous research approaches
- Lack of high-quality, comparable studies
- Highly specialized or unique research questions
- Limited comparability across studies

Tools of the Trade
Researchers use specialized software and statistical techniques like:
- Comprehensive Meta-Analysis (CMA)
- Review Manager (RevMan)
- Advanced statistical packages in R or STATA [7]
Navigating research tools can be complex. Paperguide, an AI research assistant, simplifies meta-analysis by helping researchers find, analyze, and synthesize studies with ease.
Meta-Analysis vs. Systematic Review: Understanding the Difference
Systematic Review: The Comprehensive Overview
- A structured, comprehensive summary of existing literature
- Answers a specific research question
- Follows a predefined, rigorous protocol
- Includes a detailed search and selection process
- Qualitative synthesis of research
- Does NOT necessarily include statistical combination of results
Meta-Analysis: The Statistical Deep Dive
- Goes beyond systematic review
- Statistically combines results from multiple studies
- Quantitative synthesis of research findings
- Calculates overall effect sizes
- Provides numerical summary of research outcomes
- Requires advanced statistical techniques

When to Use Each Approach
- Systematic Review:
- Limited quantitative studies
- Primarily qualitative research
- Exploring broad research landscapes
- Meta-Analysis:
- Multiple quantitative studies
- Clear, comparable research methodologies
- Need for statistical summary
- Desire to draw overarching conclusions
Supercharge Your Research with Paperguide
Feeling overwhelmed by the complexities of meta-analysis? Paperguide, the all-in-one AI research assistant designed to transform your research process.
Imagine having an intelligent research companion that can:
- Quickly locate and organize relevant research papers
- Assist in systematic literature reviews
- Help you conduct meta-analyses with advanced AI-powered tools
- Provide research-backed answers and deep insights
Why struggle alone when you can have a powerful AI research assistant by your side?
The Future of Meta-Analysis
With increasing research complexity and data availability, meta-analyses are becoming more sophisticated. They're evolving to:
- Incorporate more diverse data sources
- Use more advanced statistical techniques
- Address more complex research questions [8]
Conclusion
Meta-analysis is more than just a statistical method – it's a powerful approach to understanding scientific knowledge. By bringing together multiple studies, researchers can create a more comprehensive, reliable, and nuanced view of complex topics.
Whether you're a researcher, student, or simply curious about how scientific understanding evolves, meta-analyses offer a fascinating glimpse into the world of systematic research synthesis.
Conducting a meta-analysis doesn't have to be overwhelming. Paperguide is an AI-powered tool that transforms your research process, making meta-analysis more accessible and efficient.
Frequently Asked Questions
1. What are the different types of meta-analysis?
Quantitative, qualitative meta-synthesis, and Bayesian are the primary types—each used based on data type and research goals.
2. How is a meta-analysis different from a literature or systematic review?
A literature review summarizes; a systematic review follows a methodical process; a meta-analysis applies statistics to combine results.
3. What software is best for conducting a meta-analysis?
Popular tools include CMA, RevMan, R (meta, metafor), STATA, and AI-based tools like Paperguide.
4. Can I do a meta-analysis without advanced statistics knowledge?
Basic knowledge helps, but tools like Paperguide and RevMan simplify the process with built-in templates and guides.
5. How do I interpret a forest plot in a meta-analysis?
A forest plot visualizes effect sizes of included studies, showing individual and overall results—if the line of no effect (often 1.0 or 0) is crossed, results may not be significant.
References:
- Borenstein, M., Hedges, L. V., Higgins, J. P. T., & Rothstein, H. R. (2009). Introduction to meta-analysis. Wiley.
- Gurevitch, J., Koricheva, J., Nakagawa, S., & Stewart, G. (2018). Meta-analysis and the science of research synthesis. Nature, 555(7695), 175-182.
- Schmid, C. H., & Lau, J. (1997). Quantitative synthesis in systematic reviews. Annals of Internal Medicine, 127(9), 820-826.
- Cooper, H., Hedges, L. V., & Valentine, J. C. (2009). *The handbook of research synthesis and meta-analysis* (2nd ed.). Russell Sage Foundation.
- Song, F., Parekh, S., Hooper, L., Loke, Y. K., Ryder, J., Sutton, A. J., ... & Harvey, I. (2010). Dissemination and publication of research findings: An updated review of related biases. Health Technology Assessment, 14(8), iii, ix–xi, 1–193.
- Higgins, J. P. T., & Thompson, S. G. (2002). Quantifying heterogeneity in a meta‐analysis. Statistics in Medicine, 21(11), 1539-1558.
- Deeks, J. J., Higgins, J. P. T., & Altman, D. G. (2008). Analysing data and undertaking meta-analyses. In Higgins, J. P. T., & Green, S. (Eds.), Cochrane Handbook for Systematic Reviews of Interventions (pp. 243-296). Wiley.
- Haidich, A. B. (2010). Meta-analysis in medical research. Hippokratia, 14(Suppl 1), 29–37.