How to Use AI for Systematic Review and Meta-Analysis?
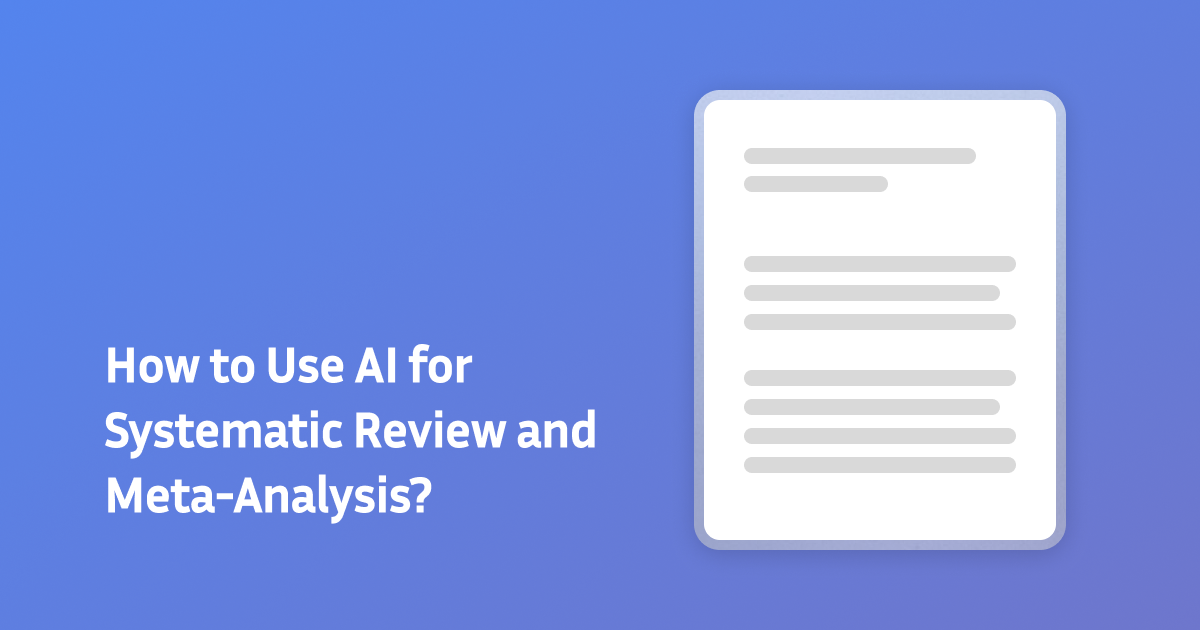
Researchers today face an overwhelming challenge: navigating through millions of academic publications to conduct comprehensive systematic reviews and meta-analyses. Traditionally, this process has been time-consuming, labor-intensive, and prone to human bias. Systematic reviews and meta-analyses are critical research methods that synthesize existing literature, but they come with significant challenges:
- Manual screening of hundreds or thousands of studies
- Risk of missing relevant research
- Inconsistent study selection
- Time-consuming data extraction
- Potential for human error and bias
Artificial Intelligence is revolutionizing this landscape, offering powerful solutions that transform how researchers conduct systematic reviews and meta-analyses. [1]
Systematic Review vs Meta-Analysis: What’s the Difference?
In short: All meta-analyses begin with a systematic review, but not all systematic reviews include a meta-analysis.
Benefits of Using AI in Systematic Review and Meta-Analysis
AI brings unprecedented advantages to research synthesis:

- Massive Time Savings
- Enhanced Accuracy
- Consistent study screening
- Reduced human bias
- Improved data extraction precision
- Comprehensive Search Capabilities
- Search multiple databases simultaneously
- Identify studies across languages
- Uncover hidden research connections
- Advanced Data Processing
- Rapid extraction of key information
- Complex statistical analysis
- Intelligent pattern recognition
- Scalability
- Handle large volumes of research
- Process studies beyond human capacity
- Continuously improve through machine learning
Step-by-Step Guide to Using AI in Systematic Reviews

1. Defining Research Questions and Protocols
AI-Powered Support:
- Generate and refine research questions
- Suggest relevant keywords
- Identify potential research gaps
- Recommend search strategies
Key Techniques:
- Natural language processing
- Semantic search algorithms
- Literature gap analysis
- Contextual keyword suggestion
2. Literature Search Automation
AI Capabilities:
- Multi-database simultaneous searching [3]
- Advanced semantic search
- Language-agnostic research discovery
- Intelligent filtering of irrelevant publications [3]
Search Optimization:
- Contextual understanding of search terms
- Synonyms and related concept identification
- Cross-referencing multiple research sources
- Minimizing manual search efforts
Paperguide Insight: Try Paperguide AI search, get research-backed answers and discover papers with semantic search.
3. Study Screening and Selection
AI-Driven Screening:
- Automated title and abstract review
- Machine learning-based inclusion/exclusion
- Consistency checking across reviewers
- Reduced screening time by up to 70%
Smart Selection Techniques:
- Adaptive learning algorithms
- Similarity matching
- Bias reduction through objective screening
- Transparent decision-making process
4. Quality Assessment
AI Quality Evaluation:
- Automated critical appraisal [4]
- Standardized assessment criteria
- Consistent evaluation across studies [4]
- Rapid identification of methodological strengths/limitations
Assessment Technologies:
- Machine learning classifiers
- Predefined quality assessment frameworks
- Comparative analysis algorithms
- Bias detection mechanisms
Paperguide Insight: Paperguide’s AI search and Literature Review provides SJR journal ranking, citation metrics for quality assessment.
5. Data Extraction and Management
AI Data Extraction Features:
- Automated information retrieval
- Structured data collection
- Reduced manual data entry
- Minimized human error
Extraction Technologies:
- Natural language processing
- Intelligent information parsing
- Automatic data structuring
- Semantic understanding of research content
With Paperguide’s Literature Review extract the complex data from papers and easily analyse in table view.
6. Statistical Analysis and Meta-Analysis
AI Analysis Capabilities:
- Advanced statistical modeling [6]
- Pattern recognition
- Automated meta-analysis techniques [6]
- Visualization generation
Analytical Features:
- Machine learning algorithms
- Statistical modeling
- Effect size calculations
- Interactive data visualization
7. Reporting and PRISMA Compliance
AI Reporting Features:
- Automated report generation
- PRISMA guideline alignment
- Interactive visualizations
- Comprehensive result summaries
Reporting Technologies:
- Natural language generation
- Adaptive reporting templates
- Visualization algorithms
- Compliance checking
Paperguide Tip: Generate professional, standardized research reports effortlessly.
Feeling overwhelmed by the research process? Paperguide, an AI research assistant, can transform your workflow. From literature searches to data synthesis, Paperguide offers intelligent tools to make your research journey smoother and more efficient.
Top AI Tools for Systematic Reviews and Meta-Analyses
1. Paperguide

- Comprehensive AI research assistant
- End-to-end research workflow support
- Advanced AI technologies
2. DistillerSR
- Systematic review management
- Collaborative screening
- Custom workflow design
3. Rayyan
- Free systematic review tool
- AI-assisted screening
- Cloud-based collaboration
4. Covidence
- Systematic review software
- Study management
- Team collaboration features
5. ASReview
- Open-source AI screening
- Active learning technology
- Research efficiency focus
6. RobotReviewer
- Automated bias assessment
- Study quality evaluation
- Machine learning algorithms
Challenges and Limitations of AI in Research
Potential Drawbacks
- Over-reliance on technology [5]
- Potential algorithmic biases [5]
- Limited contextual understanding
- Ethical considerations
Mitigation Strategies
- Human oversight
- Continuous algorithm training
- Transparent AI processes
- Interdisciplinary collaboration
Future of AI in Research Synthesis
Emerging Trends
- More sophisticated machine learning
- Enhanced natural language processing
- Greater integration of AI tools
- Improved interdisciplinary research
Ready to revolutionize your research? Paperguide offers cutting-edge AI tools designed to simplify systematic reviews and meta-analyses. Transform your research workflow with intelligent, efficient technology.
Ethical Considerations and Limitations of AI in Research
Potential Drawbacks:
- Over-reliance on AI tools without human verification
- Algorithmic bias from poorly trained models
- Lack of contextual understanding in nuanced research
- Privacy and data security concerns
Mitigation Strategies:
- Human oversight throughout the research cycle
- Transparent AI models and documentation
- Regular model validation and updates
- Ethical review and interdisciplinary collaboration
AI should assist—not replace—researchers. Critical thinking and ethical reasoning remain irreplaceable.
Frequently Asked Questions
1. Can AI fully automate a meta-analysis process?
AI cannot completely replace human expertise. It assists and accelerates the process but requires human oversight, critical thinking, and domain knowledge.
2. How does AI screen studies for inclusion in a review?
AI uses machine learning algorithms to analyze study titles, abstracts, and full texts, learning from predefined inclusion/exclusion criteria to suggest relevant studies.
3. Is AI reliable for assessing study quality and bias?
AI provides consistent, objective assessments but should be complemented by human expert review to ensure comprehensive evaluation.
4. What limitations should researchers consider when using AI?
- Potential algorithmic biases
- Limited understanding of complex context
- Need for continuous model training
- Importance of human verification
5. How do AI-based reviews compare with manual reviews in accuracy?
Studies show AI can match or exceed human accuracy in study screening, with significant time savings and reduced individual bias. [7]
Conclusion
AI is transforming systematic reviews and meta-analyses, offering researchers powerful tools to enhance efficiency, accuracy, and comprehensiveness. By embracing these technologies while maintaining critical human oversight, researchers can revolutionize their approach to literature synthesis.
Ready to elevate your research? Explore Paperguide and discover how AI can transform your systematic review workflow.
The future of research is collaborative – human intelligence working alongside artificial intelligence. Embrace these technologies, but never lose sight of the most important element: your unique scientific perspective.
References:
- Marshall, I. J., & Wallace, B. C. (2019). Toward systematic review automation: A practical guide to using machine learning tools in research synthesis. Systematic Reviews, 8(1), 163.
- O'Mara-Eves, A., Thomas, J., McNaught, J., Miwa, M., & Ananiadou, S. (2015). Using text mining for study identification in systematic reviews: A systematic review of current approaches. Systematic Reviews, 4(1), 5.
- Tsafnat, G., Glasziou, P., Choong, M. K., Dunn, A., Galgani, F., & Coiera, E. (2014). Systematic review automation technologies. Systematic Reviews, 3(1), 74.
- Thomas, J., McNaught, J., & Ananiadou, S. (2011). Applications of text mining within systematic reviews. Research Synthesis Methods, 2(1), 1-14.
- Morley, J., Floridi, L., Kinsey, L., & Elhalal, A. (2020). From what to how: An initial review of publicly available AI ethics tools, methods and research to translate principles into practices. Science and Engineering Ethics, 26(4), 2141-2168.
- Guyatt, G. H., Oxman, A. D., Vist, G. E., Kunz, R., Falck-Ytter, Y., Alonso-Coello, P., & Schünemann, H. J. (2008). GRADE: An emerging consensus on rating quality of evidence and strength of recommendations. BMJ, 336(7650), 924-926.
- Wallace, B. C., Small, K., Brodley, C. E., Lau, J., & Trikalinos, T. A. (2012). Deploying an interactive machine learning system in an evidence-based practice center: Abstrackr. Proceedings of the 2nd ACM SIGHIT International Health Informatics Symposium, 819-824.